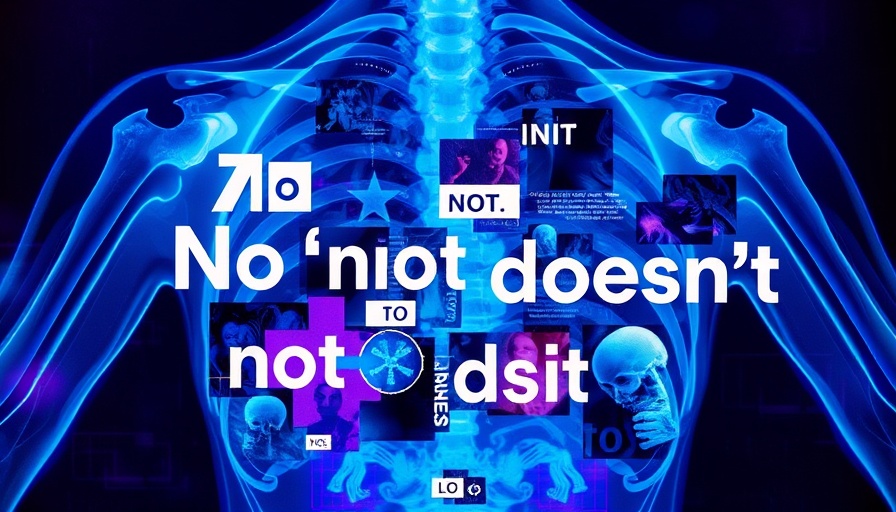
Why Vision-Language Models Struggle with Negation
Recent research has illuminated a significant gap in the capabilities of vision-language models, particularly in understanding queries embedded with negation. This fundamental limitation stems from their training mechanisms, which do not adequately equip them to interpret words such as “no” and “not.” As a result, when these AI systems encounter negations, they can produce faulty outputs, which could have dire consequences in critical applications like medical diagnostics.
The Implications of Misunderstanding Negation
The inability of these models to process negations effectively can lead to flawed interpretations in high-stakes environments. For instance, if an AI system in a healthcare setting misinterprets a negated query about a patient’s symptoms, the resulting diagnosis could overlook crucial information, impacting treatment decisions. This reality raises questions about the reliability of AI in fields where precision is paramount.
A Need for Improved Training Methods
To tackle this issue, researchers are advocating for better training methodologies that incorporate a broader range of linguistic structures. By exposing AI systems to diverse examples of negation early in their development, it may be possible to enhance their understanding and responsiveness. For innovators in AI technology, this presents an invaluable opportunity to refine models and better serve critical industries.
Future Directions for Vision-Language Models
As the field of AI continues to evolve, addressing these linguistic challenges will be essential for the advancement of vision-language technologies. Future models must not only interpret but also anticipate nuances in human language. The potential applications for contextually aware AI are immense, promising more robust solutions across a variety of sectors.
In conclusion, understanding how vision-language models interact with negation is a vital step towards developing more sophisticated AI systems. As technology advances, the integration of nuanced language processing could lead to significant improvements in both human and machine interactions.
Write A Comment